- Published on
Aggregation in MongoDB
- Authors
- Name
- Ganesh Negi
Aggregation in MongoDB
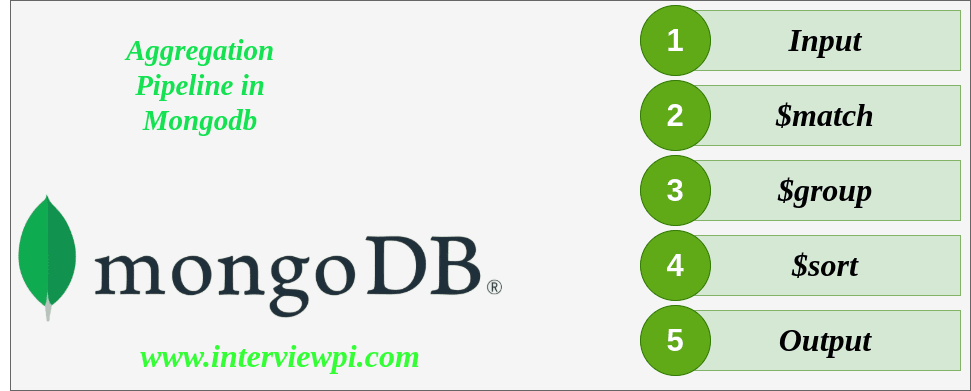
The Aggregation Framework in MongoDB is a powerful pipeline-based system designed for processing and transforming data stored within the database.
It allows developers to perform operations such as filtering, grouping, transforming, and summarizing documents to generate meaningful insights.
With its wide range of capabilities, the framework is particularly useful for handling complex data processing tasks efficiently, making it a key feature for business intelligence and analytical applications.
MongoDB provides the aggregate() method to execute aggregation operations.
This method takes an array of pipeline stages, where each stage applies a specific transformation to the data.
The output from one stage is passed as input to the next, allowing for progressive refinement of the dataset until the final result is obtained.
By structuring queries into multiple stages, MongoDB enables scalable and optimized data aggregation, making it ideal for large-scale applications.
Common Use Cases for Aggregation in MongoDB
MongoDB’s Aggregation Framework is widely used for data analysis, reporting, and data transformation.
Some common applications include:
Data Analysis: Aggregation allows you to process large datasets to identify trends and patterns, making it useful for business intelligence and analytics.
Reporting: You can generate custom reports by summarizing and structuring data in various ways.
Data Cleaning: Aggregation helps in cleaning and transforming data before inserting it into collections, ensuring data consistency and accuracy.
Key Aggregation in MongoDB Pipeline Stages
To use aggregation in mongodb effectively, you need to understand essential pipeline stages such as group, skip, and $limit.
These stages allow you to filter, transform, and manipulate data efficiently.
$match: Filters documents based on a given condition, passing only the matching documents to the next stage.
$group: Groups documents based on a specified "group key," producing a single document per unique key.
$sort: Arranges documents in a specified order before passing them to the next stage.
$skip: Skips a defined number of documents before passing the rest to the next stage.
$limit: Restricts the number of documents that proceed to the next stage, ensuring controlled data output.
By combining these pipeline stages, you can construct powerful and complex aggregations tailored to your specific data processing needs.
What is aggregation pipeline in mongodb
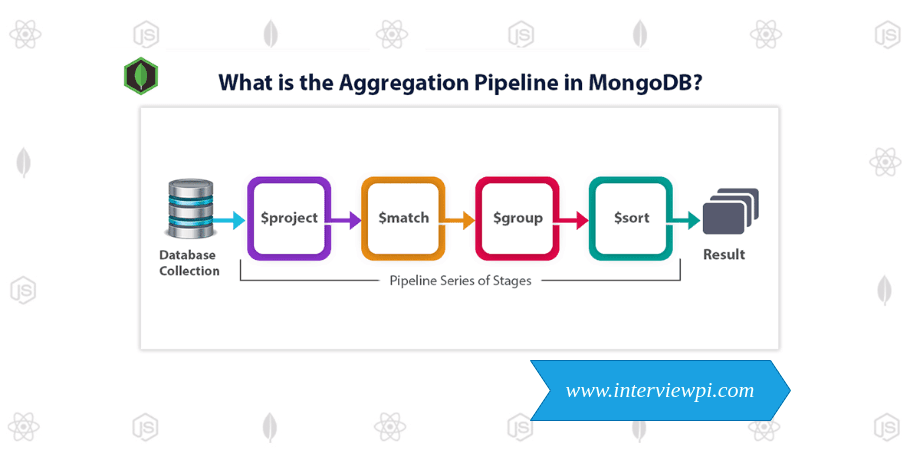
An aggregation pipeline consists of one or more stages that process documents in a sequential manner.
Each stage transforms the data in some way, and the output of one stage becomes the input for the next.
How the Aggregation Pipeline Works
Each stage performs a specific operation on the documents, such as filtering, grouping, or computing values.
The transformed documents from one stage are passed down the pipeline for further processing.
Pipelines can generate aggregated results by calculating totals, averages, maximums, minimums, and other summary metrics for groups of documents.
This structured approach allows MongoDB to efficiently process and analyze large datasets, making it a powerful tool for data analytics, reporting, and real-time insights.
Example: Aggregation Pipeline in Action
Consider an example where we want to calculate the total order quantity of medium-sized shirts, grouped by their name:
db.orders.aggregate([
// Stage 1: Filter documents for medium-sized shirts
{
$match: { size: "medium" }
},
// Stage 2: Group by shirt name and compute total quantity
{
$group: { _id: "$name", totalQuantity: { $sum: "$quantity" } }
}
])
Stage Breakdown
$match: Filters the dataset to include only documents where size is "medium".
$group: Groups the documents by the name field and sums up the quantity field to compute the total order quantity for each shirt type.
Key Aggregation Operators in MongoDB
$project - Field Selection & Transformation
- The $project stage allows for including, excluding, or modifying fields within documents. This helps reshape data for better readability.
Example: Selecting Specific Fields If we have a collection of products and want to generate a report that includes only the name and price fields, we can use $project:
db.products.aggregate([
{
$project: {
_id: 0,
name: 1,
price: 1
}
}
])
- Accumulators - Performing Calculations on Data:
- Accumulators are operators used within the $group stage to perform calculations on a set of values, producing a single result per group.
Common Accumulators:
$sum: Computes the sum of values.
$avg: Calculates the average.
$min: Returns the smallest value.
$max: Returns the largest value.
$push: Collects all values into an array.
Example: Calculating Total Sales per Category
db.sales.aggregate([
{
$group: {
_id: "$category",
totalSales: { $sum: "$amount" }
}
}
])
Essential Grouping Aggregations
- $match - Filtering Documents Used to filter documents based on specific conditions before processing them further.
Example: Filtering Sales Data for Electronics
db.sales.aggregate([
{
$match: { category: "Electronics" }
}
])
- $count - Counting Matching Documents This stage counts the number of documents that satisfy a given condition.
Example: Counting Unresolved Support Tickets
db.tickets.aggregate([
{
$match: { status: "unresolved" }
},
{
$count: "unresolvedTickets"
}
])
- $group - Grouping Documents Groups documents based on a specified field and applies an accumulator function.
Example: Total Sales by Region
db.sales.aggregate([
{
$group: {
_id: "$region",
totalSales: { $sum: "$amount" }
}
}
])
- $push - Collecting Values into an Array Used to collect multiple values into an array for each group.
Example: Gathering All Comments for Each Post
db.comments.aggregate([
{
$group: {
_id: "$postId",
comments: { $push: "$$ROOT" }
}
}
])
Working with Arrays in Aggregation
- $unwind - Expanding Array Fields into Separate Documents
The $unwind stage deconstructs arrays, creating separate documents for each element in the array.
Example: Breaking Down Orders into Individual Items
db.orders.aggregate([
{
$unwind: "$items"
}
])
This allows us to analyze sales per item rather than per order.
- $filter - Selecting Specific Elements from an Array
The $filter operator selects elements in an array that match a specific condition.
Example: Filtering Articles Tagged as "Technology"
db.articles.aggregate([
{
$match: {
tags: {
$elemMatch: {
$eq: "technology"
}
}
}
}
])
- $map - Transforming Array Elements
The $map operator modifies each element of an array based on a given expression.
Example: Converting Prices to USD
db.products.aggregate([
{
$addFields: {
pricesInUSD: {
$map: {
input: "$prices",
as: "price",
in: { $multiply: ["$$price", 1.15] } // Conversion rate
}
}
}
}
])
Enhancing Data with addFields
- $lookup - Joining Collections
The $lookup stage performs a left outer join between collections, allowing data enrichment.
Example: Joining Orders with Customer Details
db.orders.aggregate([
{
$lookup: {
from: "customers",
localField: "customerId",
foreignField: "_id",
as: "customerDetails"
}
}
])
This combines order data with customer information.
- $addFields - Adding Computed Fields
The $addFields stage allows adding new fields with computed values.
Example: Estimating Read Time for Articles
db.articles.aggregate([
{
$addFields: {
readTime: {
$divide: [
{ $subtract: ["$wordCount", 200] },
200
]
}
}
}
])
Conclusion
MongoDB’s Aggregation Framework is a powerful tool for data analysis, transformation, and reporting.
By leveraging pipeline stages like group, addFields, developers can process and analyze complex datasets efficiently.
Mastering these features allows for better data insights, optimized workflows, and enhanced application performance.